In the digital age, the realm of artificial intelligence (AI) has witnessed unprecedented advancements, reshaping industries and redefining the boundaries of what machines can achieve. At the forefront of this revolution is the concept of generative AI, a subset of AI that can generate content, be it text, images, or even music. Within this domain, a new contender has emerged, promising to change the landscape of AI-driven content creation: TruthGPT.
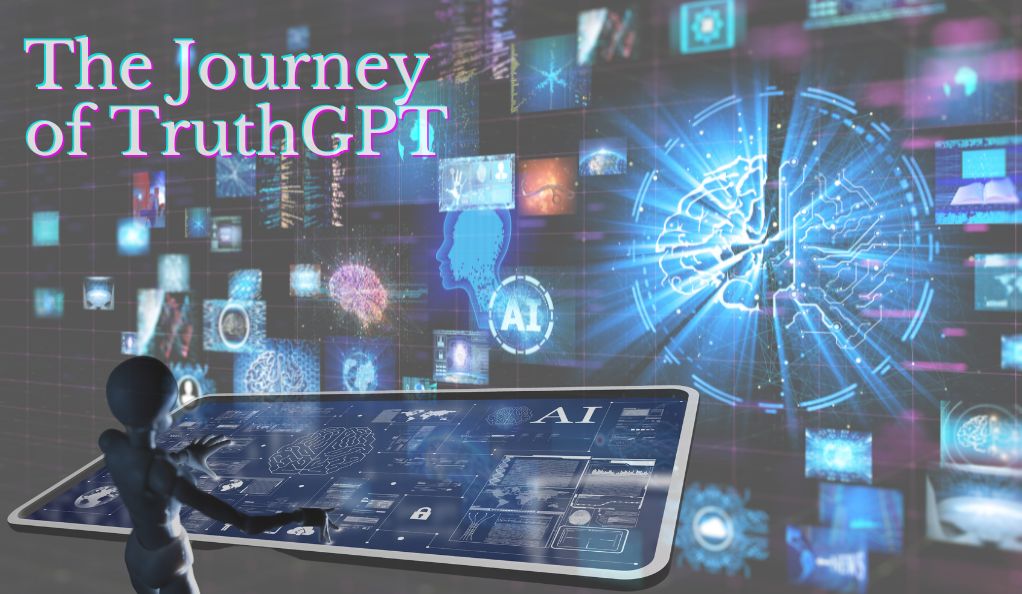
Understanding Generative AI: A Brief Overview
Generative AI operates on algorithms that allow machines to produce content. Unlike traditional AI models that are designed to make decisions based on input (like recognizing an image or translating a sentence), generative AI models create new content from scratch. They’re trained on vast datasets, learning patterns, structures, and nuances, enabling them to produce outputs that can often be indistinguishable from those created by humans.
Type of AI | Primary Function | Examples |
---|---|---|
Traditional AI | Decision-making based on input | Image recognition, voice assistants |
Generative AI | Content creation | Text generation, art creation |
The Rise of TruthGPT: Setting the Stage
TruthGPT is not just another generative AI model; it’s a vision of a truth-seeking chatbot. Proposed by tech magnate Elon Musk, this model aims to stand out by prioritizing the accuracy and truthfulness of the content it generates. In an era where misinformation can spread rapidly, the idea of an AI that seeks the truth is both revolutionary and essential.
Comparing TruthGPT with Other AI Models
AI Model | Developer | Primary Function | Unique Feature |
---|---|---|---|
ChatGPT | OpenAI | Text generation | Conversational interactions |
DeepDream | Image generation | Produces dream-like images | |
TruthGPT | Proposed by Elon Musk | Text generation | Prioritizes truthfulness and accuracy |
Why TruthGPT Matters
In the context of the digital information age, the accuracy of content is paramount. Misinformation can have real-world consequences, from influencing public opinion to causing panic. TruthGPT’s promise to seek the truth in its content generation addresses a critical need in today’s world. It’s not just about generating content; it’s about ensuring that content is accurate, reliable, and trustworthy.
The Vision Behind TruthGPT
The world of artificial intelligence has been marked by continuous evolution, with each innovation striving to address the limitations of its predecessors. Among the myriad of AI models, TruthGPT stands out, not just for its technical prowess but for the vision that fuels its creation. This vision, deeply rooted in the pursuit of truth and accuracy, is a response to the challenges of the digital age, where the line between fact and fiction can often blur.
Elon Musk: The Visionary Behind TruthGPT
Elon Musk, a name synonymous with groundbreaking technological advancements, is the driving force behind TruthGPT. Musk’s ventures, from SpaceX to Tesla, have always been characterized by pushing boundaries, and TruthGPT is no exception. His concern about the potential dangers of AI, especially in the spread of misinformation, led to the conceptualization of an AI model that prioritizes truth above all.
A Response to the AI Landscape
The current AI landscape is diverse, with models designed for various tasks, from simple data analysis to complex content creation. However, with great power comes great responsibility. As generative AI models became adept at producing human-like content, the risk of these models disseminating false or misleading information grew. TruthGPT is Musk’s answer to this challenge. It’s not just another AI model; it’s a model with a mission.
AI Model | Purpose | Potential Risks | Mitigating Features |
---|---|---|---|
ChatGPT | Conversational interactions | Spreading misinformation | Moderation and user feedback |
DeepDream | Artistic image generation | Misinterpretation of visuals | N/A |
TruthGPT | Truthful text generation | N/A | Inherent design for truth-seeking |
The Ultimate Goal: A Truth-Seeking AI
The vision behind TruthGPT goes beyond just creating content. It’s about creating content that is accurate, reliable, and trustworthy. Musk envisions TruthGPT as a tool that can help users discern fact from fiction, making it an invaluable asset in the fight against misinformation. By harnessing advanced algorithms and vast datasets, TruthGPT aims to set a new standard in AI-driven content generation, where truth and accuracy are at the forefront.
The Reliance on Vast Internet Datasets
In the realm of artificial intelligence, data is the lifeblood that fuels the capabilities of models. The quality, quantity, and diversity of this data directly influence the performance and reliability of AI systems. TruthGPT, like many of its contemporaries, relies heavily on vast internet datasets for its training and functioning. This reliance, while offering immense potential, also brings forth a set of challenges and considerations.
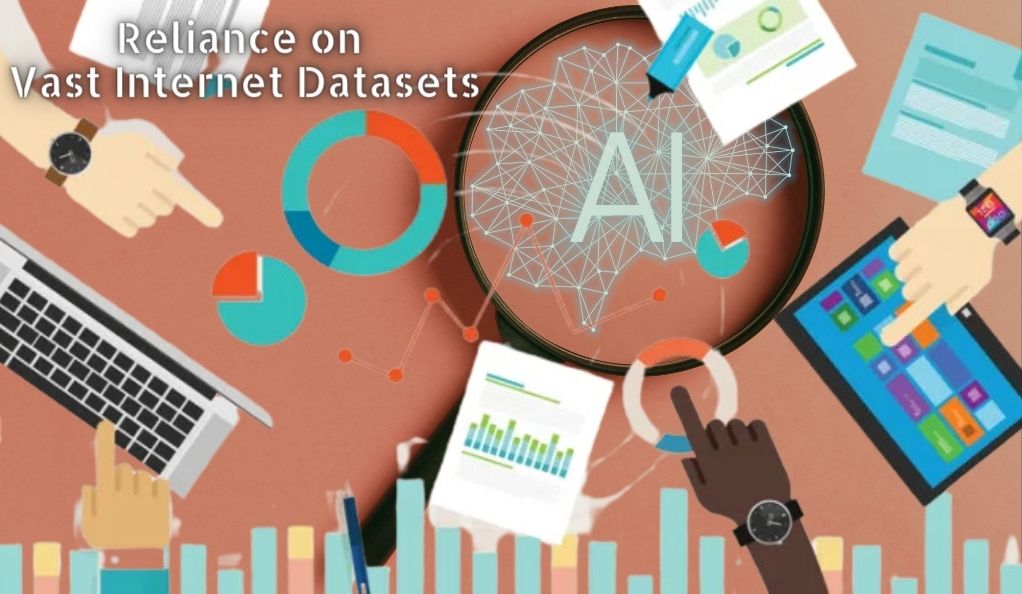
The Internet: A Treasure Trove of Data
The internet is an unparalleled repository of information, encompassing everything from scholarly articles to personal blogs, from detailed tutorials to casual conversations. For AI models, this vastness provides a rich training ground, enabling them to learn, adapt, and generate content across a myriad of topics and styles. The sheer volume of data available ensures that models like TruthGPT have a broad base to learn from, making them versatile and adaptable.
Benefits of Vast Datasets
Large datasets offer a diverse range of information, ensuring that AI models are exposed to various linguistic structures, topics, and nuances. This diversity is crucial for generative models, as it allows them to produce content that is varied, relevant, and contextually appropriate.
Advantages Description
- Diversity Exposure to a wide range of topics and styles.
- Versatility Ability to generate content across different domains.
- Adaptability Capacity to learn and evolve based on new information.
- Challenges Posed by Internet Datasets
However, the vastness of the internet also means that it’s a mix of accurate, misleading, outdated, and false information. Training an AI model on such diverse data can lead to inconsistencies in output. For a model like TruthGPT, which aims to prioritize truth, sifting through this sea of information to extract reliable data becomes a paramount challenge.
Another concern is the dynamic nature of the internet. Information is continuously updated, changed, or deleted, making it a moving target for AI training. Ensuring that the model is trained on the most recent and relevant data is a continuous task.
Striking the Balance
For TruthGPT to truly stand out as a truth-seeking AI, it must strike a balance between harnessing the vastness of internet datasets and ensuring the reliability of the data it’s trained on. This involves sophisticated filtering mechanisms, continuous updates, and rigorous validation processes to verify the accuracy of the information.
Risks of Misleading or Inaccurate Content
The digital age, characterized by the rapid dissemination of information, has brought with it a plethora of benefits. However, one of its significant challenges is the spread of misleading or inaccurate content. As AI models like TruthGPT rely heavily on vast internet datasets, understanding and mitigating the risks associated with such content becomes crucial.
The Pervasiveness of Misinformation
Misinformation is not a new phenomenon, but the internet has amplified its reach and impact. Social media platforms, blogs, forums, and even some news outlets can sometimes propagate content that is either unintentionally inaccurate or deliberately misleading. For AI models trained on these vast datasets, distinguishing between credible information and falsehoods becomes a complex task.
Implications for AI Models
When AI models ingest misleading or inaccurate content during their training phase, several issues arise:
- Output Quality: The AI might produce content that reflects the biases or inaccuracies present in its training data.
- Trustworthiness: Users might lose faith in the AI’s capabilities if it consistently delivers misleading information.
- Real-world Consequences: Inaccurate information, especially in critical areas like healthcare or finance, can have tangible negative effects.Aspect
Impact of Misleading Content
- Output Quality Degraded, biased, or factually incorrect outputs
- Trustworthiness Erosion of user trust and reliance on the AI
- Real-world Consequences Potential harm or misdirection in decision-making
The Challenge for TruthGPT
For a model like TruthGPT, which aspires to prioritize truth and accuracy, the stakes are even higher. Its very premise is to provide reliable and truthful content. Thus, ensuring that its training data is free from misleading or inaccurate content is paramount.
Mitigating the Risks
Addressing the risks of misleading content requires a multi-faceted approach:
- Data Curation: Implementing rigorous processes to curate and vet the data used for training. This might involve expert reviews, cross-referencing with trusted sources, and automated filtering mechanisms.
- Continuous Learning: Regularly updating the AI model to reflect the most recent and accurate information, ensuring it stays relevant and reliable.
- User Feedback: Incorporating feedback mechanisms where users can flag misleading outputs, allowing the model to learn and improve over time.
Legal Implications Surrounding Content Copyright
In the digital landscape, where content is both a commodity and a means of communication, the issue of copyright becomes paramount. As AI models like TruthGPT generate content, they inevitably wade into the murky waters of content copyright, raising questions about ownership, rights, and legal responsibilities.
Understanding Content Copyright
At its core, copyright is a legal concept that grants creators exclusive rights to their original works. This encompasses literary pieces, music, art, and more recently, digital content. The primary objective is to protect the creator’s intellectual property, ensuring they have control over how their work is used and distributed.
AI and Copyright: Where Does the Line Blur?
Traditional copyright frameworks were designed long before the advent of AI, making their application to AI-generated content a complex issue. Key questions arise:
- Ownership: If an AI model generates content, who owns the copyright? The developer of the AI? The user who prompted the content? Or does it remain unowned?
- Originality: Copyright typically protects original works. Can content generated by an AI, based on vast datasets of existing content, be considered original?
- Infringement: If an AI inadvertently produces content similar to existing copyrighted material, who is liable?
Issue Description
- Ownership Determining the rightful owner of AI-generated content.
- Originality Assessing the uniqueness and novelty of AI outputs.
- Infringement Addressing potential overlaps with existing copyrighted works.
TruthGPT’s Conundrum
For models like TruthGPT, which generate vast amounts of text based on extensive internet datasets, these questions are not just theoretical but practical concerns. If TruthGPT produces a piece of content that closely resembles an existing copyrighted work, the potential for legal disputes arises. Moreover, as TruthGPT aims to produce truthful and accurate content, the chances of it replicating factual information (which might be copyrighted) increase.
Navigating the Legal Labyrinth
Addressing the legal implications surrounding content copyright in the AI domain requires a multi-dimensional approach:
- Legislative Evolution: Modernizing copyright laws to accommodate the unique challenges posed by AI. This might involve creating new categories of copyright or redefining existing ones.
- Clear Licensing: AI developers can implement clear licensing agreements, specifying the rights and responsibilities of users regarding the generated content.
- Proactive Monitoring: Employing mechanisms to detect potential copyright infringements in AI outputs and rectifying them promptly.
The Intricacies of Training Data Collection
The foundation of any AI model, particularly those as advanced as TruthGPT, lies in its training data. This data not only informs the model but shapes its very essence. However, the process of collecting, curating, and implementing this data is far from straightforward. It’s a journey riddled with complexities, challenges, and ethical considerations.
The Importance of Training Data
Training data is to AI what education is to humans. It provides the foundational knowledge upon which the AI operates, learns, and evolves. The quality and diversity of this data directly influence the AI’s performance, accuracy, and reliability.
Sources of Training Data
AI models, especially those like TruthGPT, rely on a myriad of sources for their training data:
- Public Databases: Repositories that offer vast amounts of structured data on various subjects.
- Web Scraping: Extracting data from websites, forums, and other online platforms.
- User Interactions: Data generated from user inputs, feedback, and interactions with the AI.
Data Source | Advantages | Challenges |
---|---|---|
Public Databases | Structured, Reliable | Limited Diversity |
Web Scraping | Vast, Diverse | Quality Control, Ethical Concerns |
User Interactions | Real-time, Relevant | Privacy Concerns, Bias |
Challenges in Data Collection
Collecting training data is not without its hurdles:
- Volume vs. Quality: While vast amounts of data can be beneficial, ensuring its quality and relevance is crucial.
- Bias and Representation: Data can carry inherent biases, which, if not addressed, can be perpetuated by the AI.
- Ethical Considerations: Especially when scraping data or using user interactions, issues of consent, privacy, and data usage rights arise.
TruthGPT’s Approach to Training Data
For a model that prioritizes truth and accuracy, the stakes in training data collection are even higher. TruthGPT must ensure:
- Data Veracity: Ensuring the information is accurate and reliable.
- Diversity: Incorporating a wide range of sources to avoid narrow perspectives or biases.
- Continuous Updates: Regularly refreshing the training data to stay current and relevant.
Navigating the Complex Landscape
To effectively harness the power of training data, several strategies can be employed:
- Data Auditing: Regularly reviewing and assessing the data for quality, relevance, and bias.
- Ethical Guidelines: Establishing clear protocols for data collection, especially concerning user data, to ensure privacy and consent.
- Collaboration: Engaging with data scientists, ethicists, and the wider community to address challenges and continuously improve the data collection process.
Achieving Consistent Accuracy in Generative AI Systems
Generative AI systems, like TruthGPT, have revolutionized the way we interact with technology. These models can produce content, simulate scenarios, and even mimic human-like creativity. However, ensuring consistent accuracy in their outputs remains one of the most pressing challenges in the field.
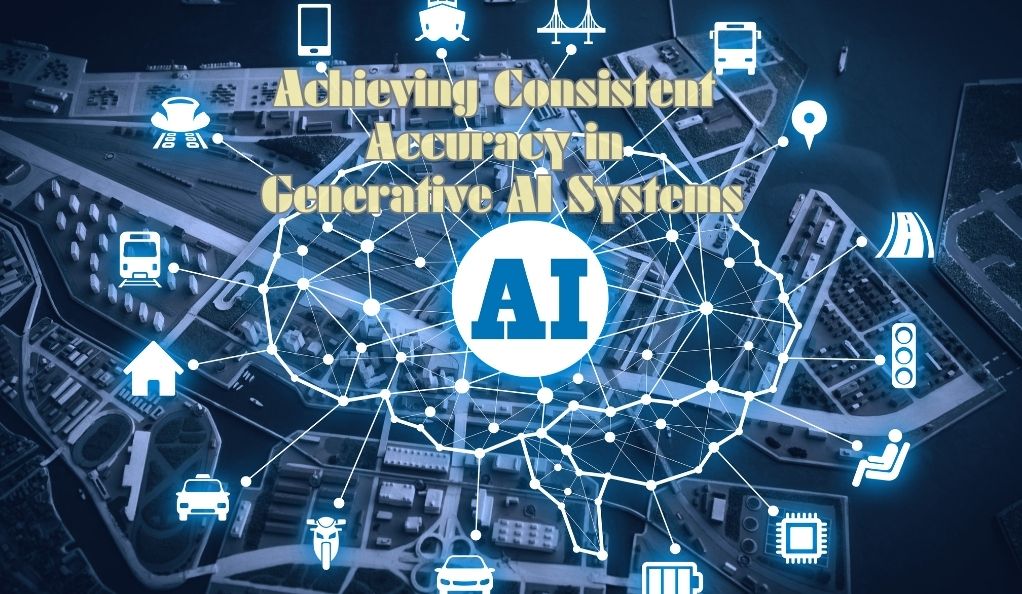
Understanding Generative AI
Generative AI operates by creating new content based on the patterns it identifies in its training data. This could range from generating text and images to simulating complex scenarios. The goal isn’t just to replicate existing data but to produce novel, coherent, and contextually relevant outputs.
The Quest for Accuracy
Accuracy in generative AI refers to the system’s ability to produce outputs that are:
- Factually Correct: Adhering to real-world truths.
- Contextually Relevant: Appropriate for the given input or scenario.
- Coherent: Logically structured and free from contradictions.
Accuracy Dimension | Description |
---|---|
Factual Correctness | Outputs align with real-world information. |
Contextual Relevance | Outputs match the given context or prompt. |
Coherence | Outputs are logically structured and consistent. |
Challenges in Ensuring Accuracy
Several hurdles stand in the way of achieving consistent accuracy in generative AI:
- Vast Training Data: The sheer volume of data can introduce noise or inaccuracies.
- Inherent Biases: Training data can carry biases, leading the AI to produce skewed outputs.
- Dynamic Information: Some information changes over time, making it challenging to stay updated.
TruthGPT’s Approach to Accuracy
For a model like TruthGPT, which emphasizes truth and reliability, the approach to accuracy is multi-faceted:
- Robust Training: Using diverse and vetted datasets to ensure a broad and accurate knowledge base.
- Feedback Loops: Incorporating user feedback to identify and rectify inaccuracies.
- Continuous Learning: Regularly updating the model with new data to stay current.
Strategies for Enhancing Accuracy
Achieving consistent accuracy in generative AI systems requires a combination of techniques:
- Data Curation: Rigorously selecting and vetting training data to ensure quality.
- Bias Mitigation: Employing algorithms and practices to identify and reduce biases in outputs.
- Real-time Validation: Cross-referencing generated content with trusted sources to verify accuracy.
Conclusion
The exploration of artificial intelligence, especially models like TruthGPT, reveals a landscape filled with potential and complexities. As we’ve navigated through the multifaceted dimensions of this technology, from data collection challenges to content generation’s legal implications, it’s clear that while AI’s capabilities are vast, they come with their own set of challenges. Generative models, trained on extensive datasets, have reshaped our technological interactions, offering the ability to produce content, simulate scenarios, and predict outcomes. However, their efficacy is deeply intertwined with the quality and diversity of their training data.
TruthGPT exemplifies the duality of generative AI, showcasing both its strengths and inherent challenges. Its dedication to accuracy and truth differentiates it, but achieving this standard is a continuous endeavor, riddled with challenges like ensuring consistent accuracy, addressing biases, and adapting to an ever-changing information ecosystem. As AI continues to evolve, ethical development, collaborative efforts across disciplines, and user empowerment will be pivotal. These elements will ensure that AI not only respects human values but also remains transparent and user-centric.
In conclusion, the AI domain, as represented by models like TruthGPT, is a fusion of promise and intricate challenges. As we further embed AI into our societal structures, our approach should be rooted in informed optimism. Recognizing its potential while proactively addressing its challenges will allow us to maximize AI’s benefits while preserving our core values and truths. As we journey further into the AI era, it’s imperative that knowledge, vigilance, and adaptability guide our path.
At bitvestment.software, our commitment is to deliver unbiased and reliable information on subjects like cryptocurrency, finance, trading, and stocks. It's crucial to understand that we are not equipped to offer financial advice, and we actively encourage users to conduct their own comprehensive research.
Read More