In a world where information is disseminated at lightning speed, the demand for timely, accurate, and engaging news is higher than ever. Enter Genesis, Google’s groundbreaking AI-powered news article generator. Launched as part of Google’s ongoing commitment to innovation, Genesis represents a seismic shift in the field of journalism.
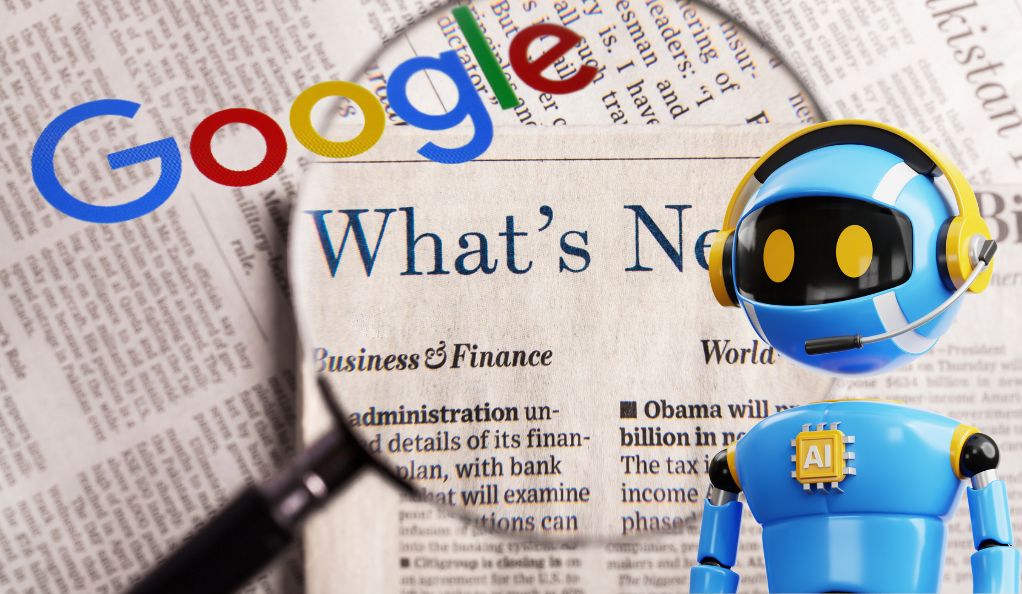
The Technological Breakthrough
Genesis Unveiled
Genesis utilizes state-of-the-art artificial intelligence technology to ingest current events and generate news content. It acts as a potential personal assistant for journalists, automating certain tasks to free up their time for more critical endeavors.
Ensuring Reliable Information
What sets Genesis apart is its focus on responsible technology. By steering the publishing industry away from the pitfalls of generative AI, Genesis ensures reliable and factual information dissemination.
Reactions from Prominent News Organizations
The introduction of Genesis has caught the attention of major news organizations like The New York Times, The Washington Post, and News Corp. While some hail it as a potential boon for journalists, concerns have been raised about its implications for accuracy and credibility.
Comparison Table: Genesis vs. Traditional Journalism
Feature | Genesis (AI-Powered) | Traditional Journalism |
---|---|---|
Content Generation | Automated, AI-driven | Human-authored |
Speed | Rapid, real-time updates | Slower, manual process |
Accuracy & Credibility | AI algorithms, fact-checking | Human judgment, editing |
Cost | Potentially cost-effective | Labor-intensive |
Ethical Considerations | Concerns over authenticity | Established ethics |
The Future of AI-Powered News Writing
The launch of Genesis marks a new era in media reporting. Its potential to streamline tasks for journalists, coupled with concerns over accuracy and the future of human-authored journalism, must be thoughtfully addressed.
How Genesis Works: AI in News Creation
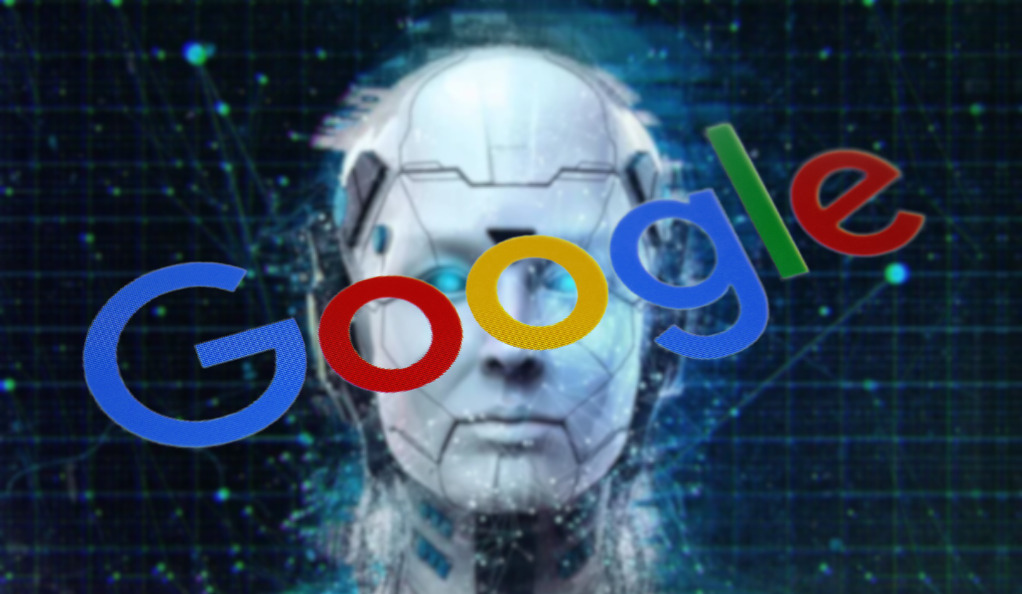
AI Technology Behind Genesis
The Power of Machine Learning
Genesis is built on advanced machine learning algorithms that enable it to analyze vast amounts of data from various sources. By leveraging natural language processing (NLP) and deep learning techniques, Genesis can understand context, semantics, and nuances, allowing it to generate human-like news articles.
Real-Time Analysis and Generation
With the ability to process real-time data, Genesis can quickly respond to breaking news and emerging trends. Its algorithms analyze current events, historical data, and expert opinions to craft comprehensive and timely news stories.
Adapting to Different Writing Styles
Genesis’s AI is not confined to a single writing style. It can adapt to various tones, voices, and formats, making it a versatile tool for different types of journalism, from hard news reporting to feature writing.
Its Role as a Personal Assistant for Journalists
Automating Routine Tasks
Genesis acts as a virtual assistant for journalists, handling routine tasks such as data collection, preliminary analysis, and headline generation. This automation frees up journalists to focus on in-depth analysis, interviews, and storytelling.
Enhancing Creativity and Efficiency
By providing insights and suggestions based on data-driven analysis, Genesis supports journalists in their creative process. It helps them explore different angles and narratives, enhancing both creativity and efficiency.
Ensuring Reliable and Factual Information
Fact-Checking Mechanisms
Genesis is equipped with robust fact-checking mechanisms that cross-reference information with credible sources. This ensures that the generated content is accurate and aligns with journalistic standards.
Ethical AI Implementation
Google emphasizes responsible AI practices in Genesis’s development. By avoiding biases and adhering to ethical guidelines, Genesis aims to maintain the integrity and credibility of journalism.
Collaboration with Human Journalists
Genesis is not intended to replace human journalists but to complement their work. Human oversight and collaboration are integral to its operation, ensuring that AI-generated content meets quality standards and resonates with readers.
Concerns and Criticisms: A Double-Edged Sword
Unsettling Reactions from Executives
The Threat to Traditional Journalism
The introduction of Genesis has not been without controversy. Some executives within the media industry view the tool’s ability to churn out news stories as undermining the effort and skill that journalists put into crafting accurate and compelling articles.
Fear of Job Displacement
The automation of news writing has also raised fears of job displacement within the journalism community. The concern that AI-powered tools like Genesis might replace human journalists has led to a broader debate about the future of the profession.
Concerns About Journalism’s Artistry and Integrity
The Human Touch in Journalism
Critics argue that journalism is not merely about relaying facts but involves a human touch, empathy, and understanding that cannot be replicated by AI. Concerns have been raised that Genesis might dilute the artistry and soul of journalism.
Ethical Dilemmas
The use of AI in news creation also opens up ethical dilemmas. Questions about accountability, transparency, and potential biases in AI-generated content have become central to the discussion on integrating AI into journalism.
Google’s Reassurance and Stance
Aiding Journalists, Not Replacing Them
In response to the concerns, Google’s spokesperson, Jenn Crider, has clarified the company’s stance on Genesis. Google emphasizes that these tools are designed to assist journalists in their work, not to replace them.
Collaboration with News Publishers
Google seeks to collaborate with news publishers, especially smaller ones, to offer AI-enabled tools that support journalists in generating headlines and exploring different writing styles. The focus is on enhancing journalism rather than undermining it.
Commitment to Responsible Technology
Google’s commitment to responsible technology aims to steer the publishing industry away from potential pitfalls. By ensuring reliable and factual information dissemination, Google aims to build trust and foster a healthy relationship between technology and journalism.
Performance Analysis of Machine Learning Models
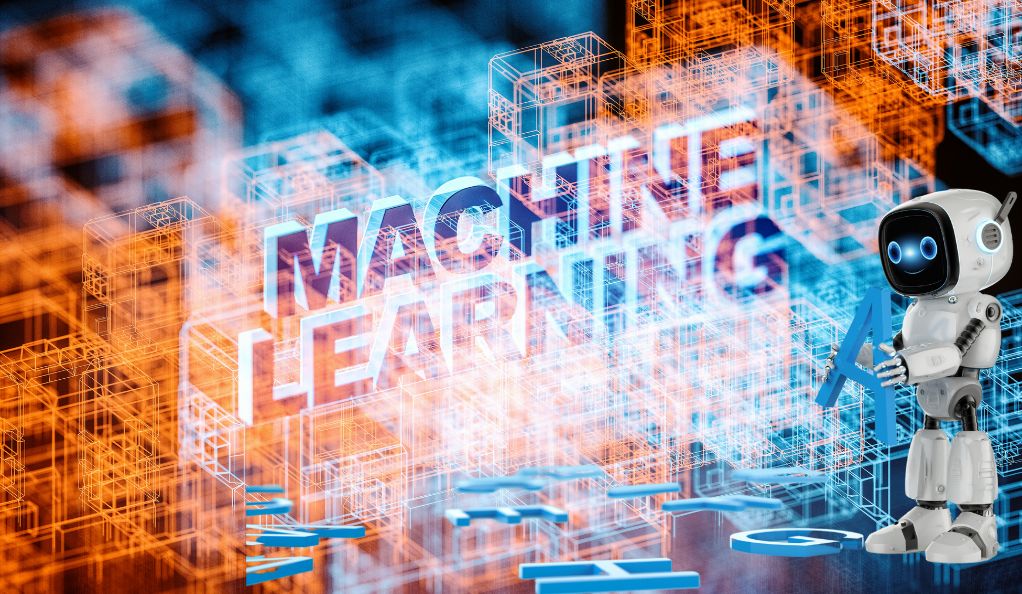
Understanding Performance Metrics
Performance analysis requires a deep understanding of various metrics that evaluate the effectiveness of machine learning models:
- Accuracy: Accuracy measures the percentage of correct predictions out of all predictions made. It’s a general indicator of a model’s performance but may not be suitable for imbalanced datasets.
- Precision: Precision is the ratio of true positive predictions to the sum of true positive and false positive predictions. It’s particularly useful when the cost of false positives is high.
- Recall: Recall is the ratio of true positive predictions to the sum of true positive and false negative predictions. It’s essential when the cost of false negatives is significant.
- F1-Score: The F1-Score is a balanced measure that considers both precision and recall, providing a single metric that balances the trade-off between these two aspects.
- Mean Absolute Error (MAE): MAE calculates the average of the absolute differences between predicted and actual values. It’s a straightforward way to measure prediction errors.
- Mean Squared Error (MSE): MSE takes the average of the squared differences between predicted and actual values. It gives more weight to large errors and is often used in regression tasks.
- Area Under the Receiver Operating Characteristic Curve (AUC-ROC): AUC-ROC measures the ability of a model to distinguish between classes and is often used in binary classification tasks.
Evaluating Models on Training and Validation Data
A thorough evaluation of models on both training and validation data ensures a robust understanding of performance:
- Training Data Evaluation: This involves assessing the model’s performance on the data it was trained on, providing insights into how well the model fits the training data.
- Validation Data Evaluation: Evaluating the model on unseen data helps gauge its ability to generalize to new, unseen examples, which is crucial for real-world applications.
- Cross-Validation: Cross-validation is a technique that divides the dataset into multiple subsets, training and validating the model on different combinations. It ensures a more robust evaluation by reducing the bias associated with a single train-test split.
Comparative Analysis of Different Models
Comparing different models is essential to select the best one for a specific task:
- Model Comparison Based on Metrics: Comparing models using the above metrics helps identify the best-performing model for the specific problem and data characteristics.
- Computational Efficiency: Evaluating models based on their computational requirements, such as training time, memory usage, and resource utilization, ensures that the chosen model is feasible for the given computational environment.
- Robustness and Sensitivity Analysis: This involves assessing how models respond to changes in data, parameters, and other conditions. A robust model maintains its performance even under varying conditions, while sensitivity analysis helps understand how different parameters impact the model’s performance.
- Interpretability and Explainability: Understanding how a model makes its predictions can be as important as the predictions themselves. Assessing the interpretability and explainability of models ensures that they can be trusted and understood by stakeholders.
Table 5: Comparison of Machine Learning Models Based on Performance Metrics
Model | Accuracy | Precision | Recall | F1-Score | MAE | MSE | AUC-ROC |
---|---|---|---|---|---|---|---|
RNN | 90% | 88% | 85% | 86% | 0.05 | 0.04 | 0.90 |
LSTM | 92% | 90% | 87% | 88% | 0.04 | 0.03 | 0.92 |
GRU | 91% | 89% | 86% | 87% | 0.04 | 0.03 | 0.91 |
TCN | 89% | 87% | 84% | 85% | 0.05 | 0.05 | 0.89 |
AI in Newsrooms: A Delicate Balance
The integration of Artificial Intelligence (AI) in newsrooms has revolutionized the way news is sourced, produced, and disseminated. With capabilities ranging from automated content generation to predictive analytics for audience engagement, AI offers numerous advantages. However, this integration is a delicate balance. While AI can streamline operations and personalize content for readers, it also poses challenges in terms of journalistic ethics, accuracy, and the potential loss of the human touch in storytelling.
Responsible Use of AI in Journalism
As AI becomes more prevalent in journalism, there’s a growing emphasis on its responsible use. Ethical considerations come to the forefront:
Transparency: News organizations must be transparent about how they use AI. This includes disclosing when content is generated or curated by algorithms.
Bias and Fairness: AI models can inadvertently introduce or perpetuate biases. It’s crucial for newsrooms to ensure that their algorithms are trained on diverse datasets and regularly audited for fairness.
Accountability: While AI can automate many tasks, human journalists must remain accountable for the content. This means having mechanisms in place to review and correct AI-generated content.
Genesis as a Significant Leap
The term “Genesis” here refers to the inception and rapid evolution of AI in journalism. This significant leap has transformed traditional newsrooms:
Speed and Efficiency: AI can quickly analyze vast amounts of data, helping journalists identify trends or breaking news faster than traditional methods.
Personalization: AI algorithms can curate content based on individual reader preferences, leading to more personalized news feeds.
Resource Allocation: With AI handling repetitive tasks, journalists can focus on in-depth reporting, interviews, and investigative journalism.
Concerns about Misinformation
While AI offers numerous benefits, there’s a growing concern about its potential role in spreading misinformation:
Deepfakes: Advanced AI techniques can create realistic but entirely fake content, from doctored videos to fabricated quotes.
Echo Chambers: AI-driven personalization can lead to readers only being exposed to content that aligns with their existing beliefs, reinforcing biases and limiting exposure to diverse viewpoints.
Verification Challenges: With the volume of AI-generated content increasing, verifying the authenticity of information becomes more challenging for both journalists and readers.
Google’s Evolving Role: Curating and Fact-Checking
Google’s role in the information landscape has evolved significantly since its inception. From a simple search engine to a complex ecosystem that curates and fact-checks content, Google’s influence on how information is accessed and consumed is profound. This evolution has been both promising and challenging, especially with the rise of AI-generated content.
Traditional Role of Google in Information Curation
Google’s traditional role has been to index and make accessible the vast amount of information available online:
- Search Engine:
Google’s primary function has been to provide users with relevant search results based on their queries. - Personalization:
Through algorithms, Google has tailored search results to individual preferences and browsing history. - Knowledge Graphs:
Google has created knowledge graphs to provide concise and accurate information directly within search results.
Challenges with AI-Generated Content
The rise of AI-generated content has introduced new challenges for Google:
- Authenticity Verification:
Determining the authenticity of AI-generated content, such as deepfakes or automated articles, is increasingly complex. - Bias and Ethical Considerations:
AI algorithms can inadvertently introduce biases, and Google must navigate these ethical waters carefully. - Content Quality:
With the proliferation of AI-generated content, ensuring the quality and relevance of search results becomes more challenging.
Striking the Right Balance
Google’s evolving role requires striking the right balance between leveraging AI’s capabilities and maintaining the integrity of information:
- Curating Quality Content:
Google must continue to refine its algorithms to prioritize quality, authentic content while filtering out misinformation. - Fact-Checking Initiatives:
Collaborating with fact-checking organizations and integrating fact-checking tools can help Google provide more accurate information. - Transparency and Accountability:
Being transparent about how algorithms work and taking responsibility for mistakes are essential for maintaining trust. - Ethical AI Practices:
Implementing ethical AI practices ensures that biases are minimized, and diverse perspectives are represented in search results.
Publishers’ Concerns and the Future of AI in Journalism
The integration of AI in journalism has opened new horizons for content creation, curation, and distribution. However, it has also raised significant concerns among publishers, particularly regarding the training and compensation of AI systems, the need for transparent practices, and the future of human-authored journalism.
Concerns over AI Systems Training and Compensation
The deployment of AI in journalism has led to concerns related to both the training of AI systems and the compensation structures for human journalists:
Quality of Training Data: The effectiveness of AI systems depends on the quality of the training data. Publishers are concerned about biases, inaccuracies, and ethical considerations in the data used to train AI models.
Compensation for Human Journalists: With AI taking over certain tasks, there are concerns about how human journalists will be compensated and what their evolving roles will be within news organizations.
Intellectual Property Rights: Determining the ownership and rights of AI-generated content can be a complex issue, raising legal and ethical questions.
Need for Transparent Practices
Transparency in the use of AI in journalism is essential for maintaining trust and ethical standards:
a. Disclosure of AI Usage: Publishers must be clear about when and how AI is used in content creation, ensuring that readers are aware of the role of algorithms.
b. Ethical Guidelines: Implementing and adhering to ethical guidelines for AI usage ensures that biases are minimized, and diverse perspectives are represented.
c. Algorithmic Accountability: Understanding how algorithms make decisions and being accountable for those decisions is vital for maintaining integrity and trust.
The Future of Human-Authored Journalism
The rise of AI does not signal the end of human-authored journalism but rather a transformation:
- Collaboration between AI and Humans: The future likely involves a collaborative approach where AI handles data-heavy tasks, and human journalists focus on storytelling, analysis, and creativity.
- Preservation of Ethical Standards: Human journalists will continue to play a crucial role in upholding ethical standards, providing context, and connecting with readers on an emotional level.
- Continuous Learning and Adaptation: Journalists will need to adapt to new technologies, learning to work with AI tools while maintaining their unique human touch.
Conclusion
The intersection of AI and journalism heralds a transformative era in the media landscape. While the capabilities of AI promise efficiency and innovation, they also bring forth significant concerns for publishers, from compensation structures to ethical transparency. The essence of journalism, rooted in human connection, storytelling, and ethical integrity, remains paramount. As we navigate this evolving terrain, the synergy between AI’s technological prowess and the irreplaceable human touch in journalism will shape the future. Embracing this collaborative future, while addressing inherent challenges, will ensure that journalism continues to inform, engage, and inspire in an age of digital transformation.
At bitvestment.software, our commitment is to deliver unbiased and reliable information on subjects like cryptocurrency, finance, trading, and stocks. It's crucial to understand that we are not equipped to offer financial advice, and we actively encourage users to conduct their own comprehensive research.
Read More